Segmentation of Seabed Sediment Images Based on Convolutional Neural Network
DOI:
https://doi.org/10.32908/JMEE.v11.2024082601Keywords:
Sediments, Particle size, U-Net, Spatial attention modules, Image segmentationAbstract
The analysis of particle sizes in seabed sediments plays an important role in various fields. However, acquiring digital sediment images has been challenging due to the characteristics of sediment particles and the complexity of the seabed exploration environment. The goal of this study is to address the issue of particle segmentation in sediment images and propose an innovative network called SAMU-Net. Innovations are as follows. Firstly, SAMU-Net combines spatial attention modules with the traditional U-Net model, which allows for contextual information extraction and adaptive perception of feature variations. Secondly, the model adopts the idea of multi-scale fusion, enabling networks to better understand the structural and semantic information of the images by fusing feature maps at different scales. In addition, using the drop blocks and normalization, we speed up the training convergence of the model and avoid the overfitting phenomenon. The results show that the segmentation results predicted by the SAMU-Net network are highly similar to the ground truth and have a better segmentation performance compared to the existing algorithms. At the same time, it provides a new solution idea to uneven illumination and other noise interference problems in the digital imaging information of sediments.
References
Alom, M. Z., Yakopcic, C., Hasan, M., et al. (2019). Recurrent residual U-Net for medical image segmentation. Journal of Medical Imaging, 6(1), 014006-014006.
Chen, L. C., Papandreou, G., Schroff, F., et al. (2017). Rethinking atrous convolution for semantic image segmentation. Journal Title, volume, pages.
Chen, T., Xie, J., Cai, H. (2020). Improved Convolutional Neural Network Fault Diagnosis Method Based on Dropout. In Proceedings of the 7th International Forum on Electrical Engineering and Automation (IFEEA), 2020.
Fu, J., Liu, J., Tian, H., et al. (2019). Dual attention network for scene segmentation. In Proceedings of the IEEE/CVF conference on computer vision and pattern recognition, 2019, 3146-3154.
Guo, C., Szemenyei, M., Pei, Y., Yi, Y., & Zhou, W. (2019). SD-Unet: A Structured Dropout U-Net for Retinal Vessel Segmentation. 2019 IEEE 19th International Conference on Bioinformatics and Bioengineering (BIBE), Athens, Greece, pp. 439-444.
Guo, C., Szemenyei, M., Yi, Y., Xue, Y., Zhou, W., & Li, Y. (2020). Dense Residual Network for Retinal Vessel Segmentation. ICASSP 2020 - 2020 IEEE International Conference on Acoustics, Speech and Signal Processing (ICASSP), Barcelona, Spain, pp. 1374-1378.
Guo, C., Szemenyei, M., Yi, Y., et al. (2021). SA-Unet: Spatial attention u-net for retinal vessel segmentation. In Proceedings of the 25th International Conference on Pattern Recognition (ICPR), IEEE, 2021, 1236-1242.
Ghiasi, G., Lin, T. Y., Pang, R., & Le, Q. V. (2019). NAS-FPN: Learning scalable feature pyramid architecture for object detection. In The IEEE Conference on Computer Vision and Pattern Recognition (CVPR), June 2019.
Ji, Y., Li, Z., Li, Y., et al. (2021). An Improved CNN Algorithm Based on Batch Normalization and Dropout for Electricity Load Forecasting. Sustainability, 13(8), 4265.
Liu, D., Li, J., Yuan, Q. (2021). A spectral grouping and attention-driven residual dense network for hyperspectral image super-resolution. IEEE Transactions on Geoscience and Remote Sensing, 59(9), 7711-7725.
Marc, O., Turowski, J. M., Meunier, P. (2021). Controls on the grain size distribution of landslides in Taiwan: the influence of drop height, scar depth and bedrock strength. In Proceedings, 2021, 995-1011.
Oktay, O., Schlemper, J., Folgoc, L. L., et al. (2018). Attention U-Net: Learning where to look for the pancreas. Journal Title, volume, pages. (arXiv preprint arXiv:1804.03999)
Ronneberger, O., Fischer, P., Brox, T. (2015). U-net: Convolutional networks for biomedical image segmentation. In Medical Image Computing and Computer-Assisted Intervention–MICCAI 2015: 18th International Conference, Munich, Germany, October 5-9, 2015, Proceedings, Part III, volume 18, 234-241. Springer International Publishing.
Wang, X., Tang, X., Yang, Z., et al. (2020). Normalized window size based CLAHE algorithm. Journal of Beijing University of Posts and Telecommunications, 43(02), 85-92.
Wang, G., Li, Y., Wei, H., et al. (2021). Automated Segmentation of Retinal Blood Vessels Using an Improved U-Net Based on Attention Mechanism with B-CNN Model. Journal of Healthcare Engineering, 2021, 6639912.
Yan, Z., Zhu, D., Guo, L., et al. (2021). A general evaluation metric for image segmentation using a novel shape similarity measure. Computer Vision and Image Understanding, 204, 103091.
Zhou, Z., Siddiquee, M. M. R., Tajbakhsh, N., et al. (2018). UNet++: A Nested U-Net Architecture for Medical Image Segmentation. DOI: 10.1007/978-3-030-00889-5_1.
Zhou, Z., Siddiquee, M., Tajbakhsh, N., et al. (2019). Unet++: Redesigning skip connections to exploit multiscale features in image segmentation. IEEE Transactions on Medical Imaging, 39(6), 1856-1867.
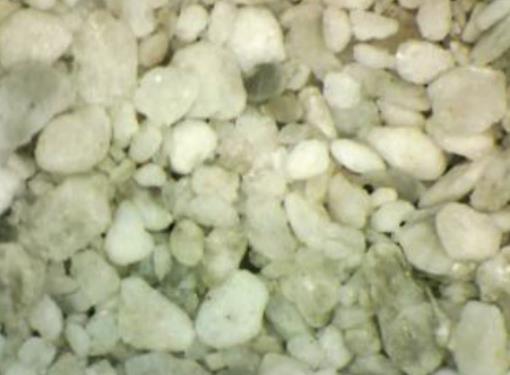